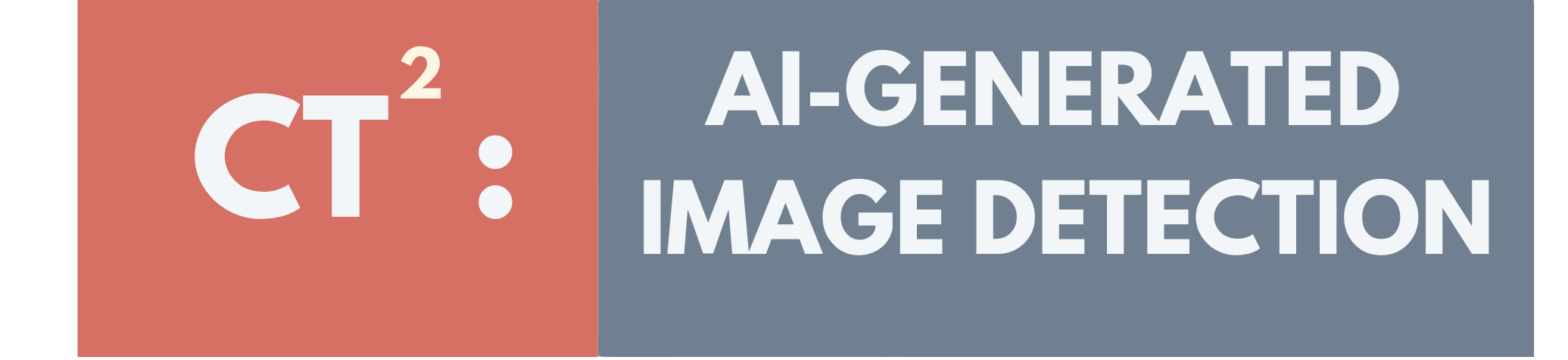
@ AAAI 2025
Fourth Workshop on ​Multimodal Fact Checking and Hate Speech
Detection
February, 2025
About : CT2 - AI Generated Image Detection
Important News Datasets Released:
CT2 - AI Generated Image Detection
- Deadline Extended to 14th December 2024
- Check the leaderboard: Leaderboard
- Fill the form to submit your scores to the leaderboard: Leaderboard Form
- Updated Test Dataset Shared on 5th December. Kindly fill the form to get access to the drive link and dataset.
- Colab: https://codalab.lisn.upsaclay.fr/competitions/20331
- Form: https://forms.gle/nmKYsCYY2odZGP4x9
Dataset:
Several text-to-image generation systems are available today. To determine which types of model-generated images are easier or harder to detect, we will include a diverse range of models, such as Stable Diffusion 3 (SD 3), Stable Diffusion XL (SDXL), Stable Diffusion 2.1 (SD 2.1), DALL-E 3, and Midjourney 6. We will release a dataset of 50K samples for this task, developed based on the MS COCO dataset.
The process involves taking the original MS COCO captions and images, feeding the captions into each of the models (SD 3, SDXL, SD 2.1, DALL-E 3, and Midjourney 6), and storing the generated images. This resulting dataset will be referred to as MS COCOAI. A snapshot of the data can be viewed here.
Tasks:
- Task A: This is a binary classification task where the goal is to determine whether each given image was generated by AI or created by a human.
- Task B: Building on Task A, this task challenges participants to determine which specific model generated a given image. Participants must identify whether the images were produced by models such as SD 3, SDXL, SD 2.1, DALL-E 3, or Midjourney 6.
Baseline:
AI-generated image detection has recently garnered significant research attention. Proposed detection techniques can be broadly categorized into two paradigms: artifact-based detection and feature representation-based detection.
For artifact-based detection, techniques include NPR, DM Image Detection, GAN DCT Analysis, and more. For feature representation-based detection, examples include CNN Detection, DIRE, AEROBLADE, LASTED, and others.
As generative models become increasingly advanced, the need for sophisticated detection methods is growing. Studies have observed that each generative model leaves distinct traces in the frequency domain of the generated images, which can be considered an artificial fingerprint used for detection.
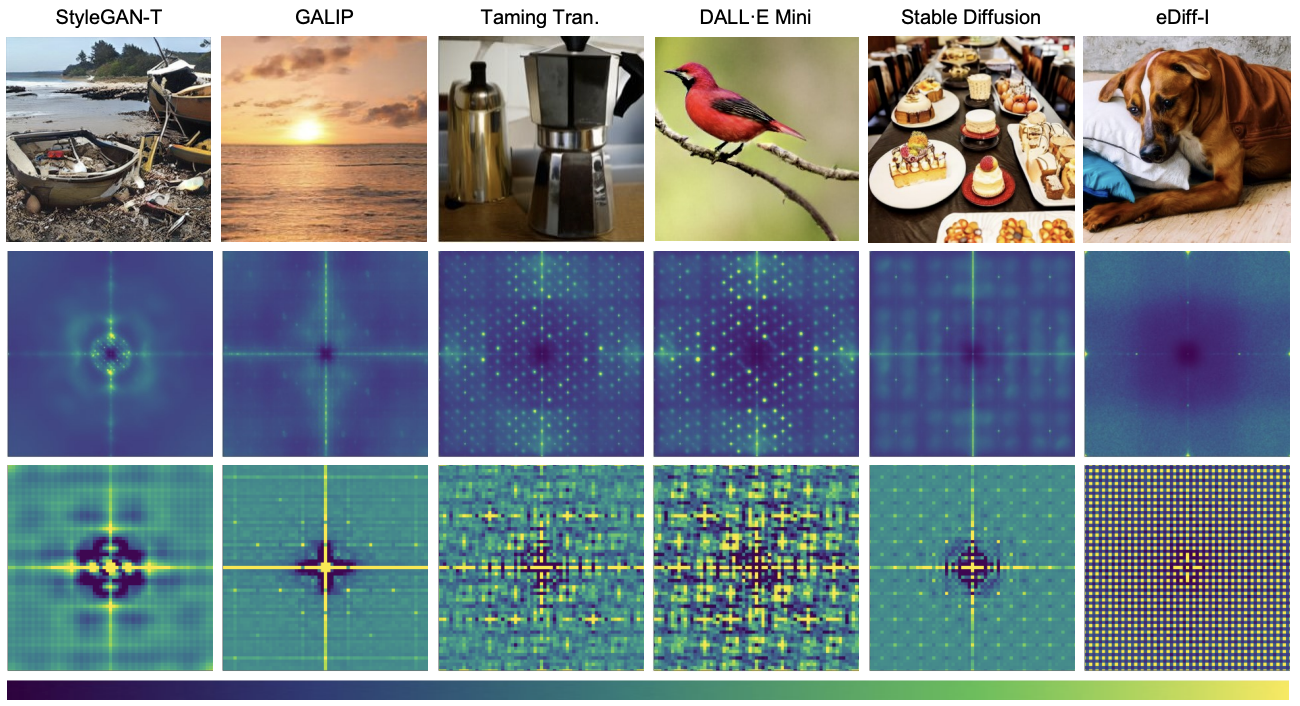
Synthetic images generated by models like StyleGAN-T, GALIP, Taming Transformers, DALL·E Mini, Stable Diffusion, and eDiff-I (top) contain unique traces known as artificial fingerprints. These fingerprints are detectable in the frequency domain as spectral peaks in the power spectra (middle) and in the spatial domain as anomalous patterns in the auto-correlation (bottom). Similar artifacts are observed in models sharing similar architectures, enabling forensic analysis of generated content.
According to a report by the European Union Law Enforcement Agency, 90 percent of online content could be synthetically generated by 2026. Generative systems like DALL-E and Stable Diffusion are impressive but raise concerns about potential misuse, particularly in spreading misinformation. Some examples can be seen below:
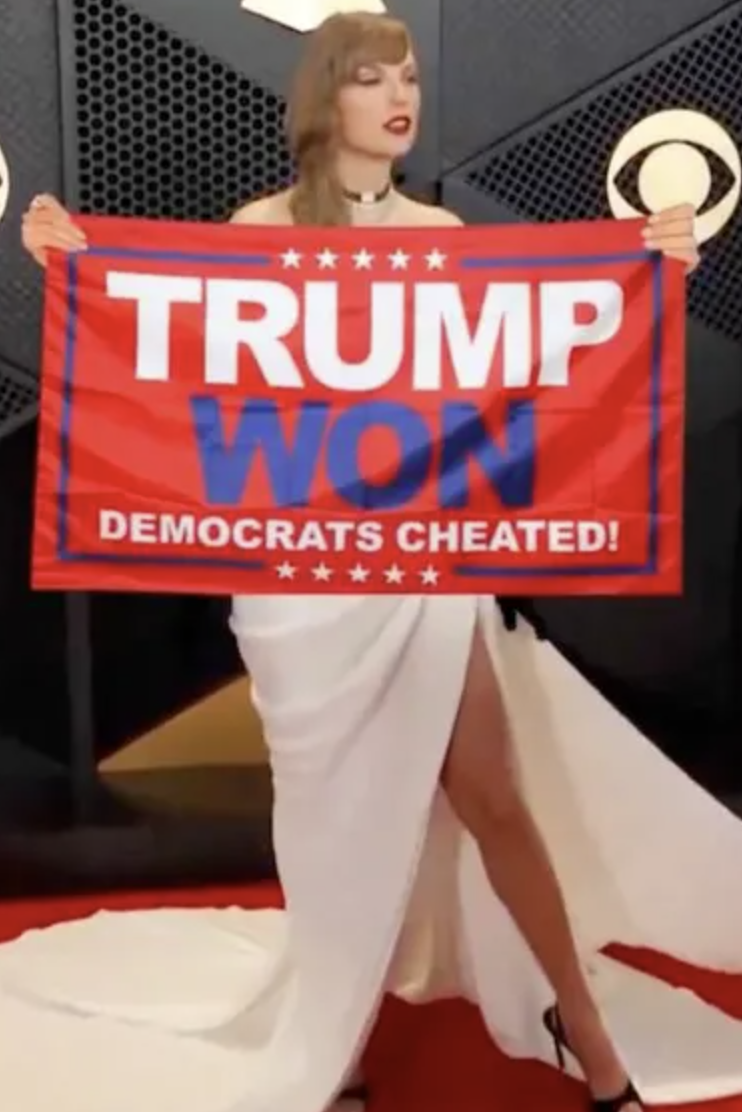
A screenshot from a video showing Taylor Swift holding a flag reading "Trump Won" went viral in February 2024. The video, shared by numerous accounts on X, has reached over 4.5 million viewers. However, it is important to note that this content is AI-generated. For more details, see the Forbes story.
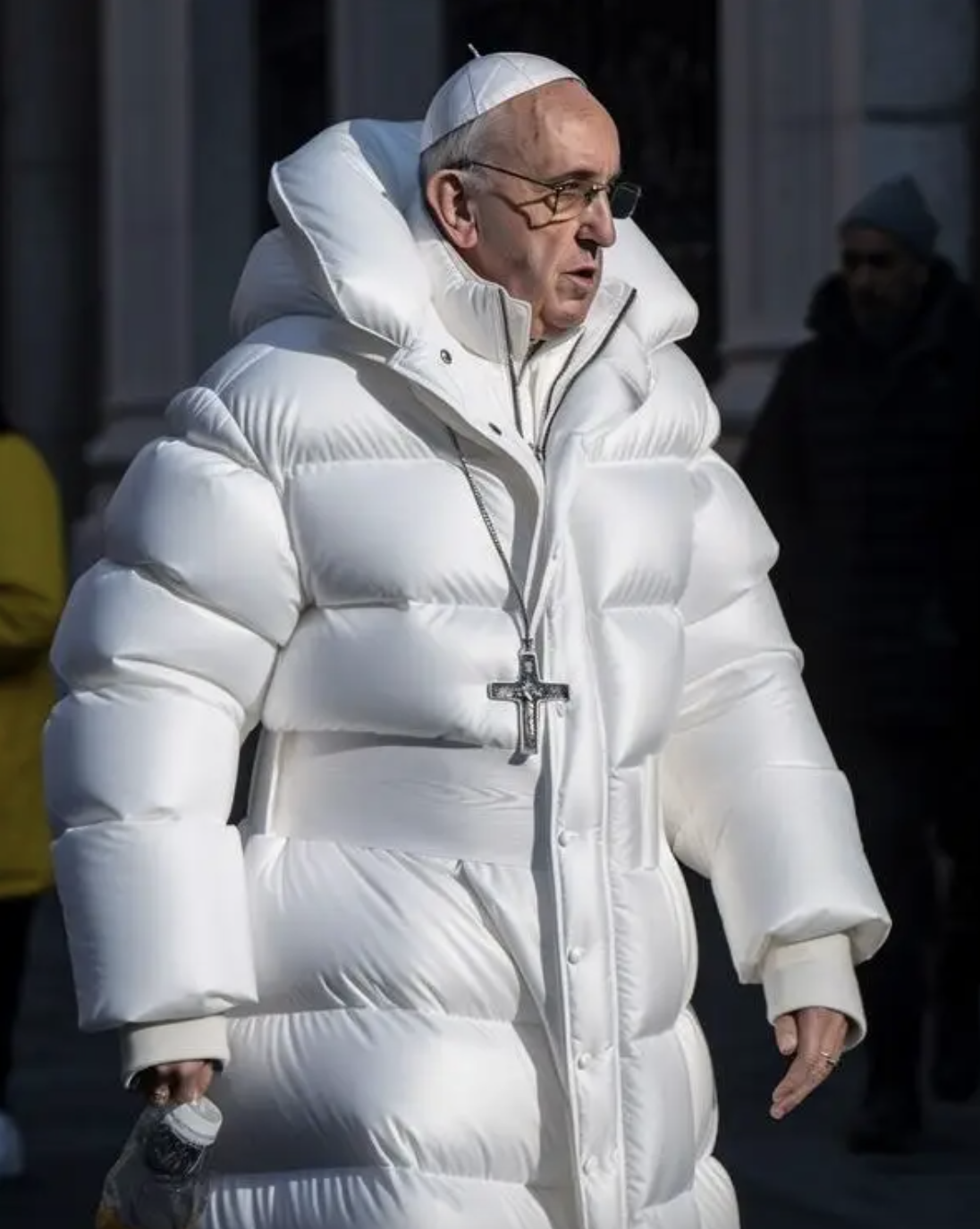
An AI-generated image of Pope Francis wearing a gigantic white puffer jacket went viral on social media platforms like Reddit and Twitter (X) in March of last year. This image sparked widespread media discussions about the potential misuse of Generative AI technologies, ultimately becoming an iconic example of AI-generated misinformation. For more details, see the Forbes story.
Leaderboard
S.No | Name | Team Name | Score for Task-A ▲▼ | Score for Task-B ▲▼ |
---|---|---|---|---|
1 | Suril | SeeTrails | 0.8334 | 0.4986 |
2 | Duong Anh Kiet | dakiet | 0.833 | 0.4935 |
3 | TSAN-TSUNG | NYCU | 0.8329 | 0.491 |
4 | Shaurya | random.py | 0.8326 | 0.4936 |
5 | Xiaoyu | Xiaoyu | 0.8316 | 0.4888 |
6 | Raghad_Khalid | TAHAKOM | 0.8305 | 0.4816 |
7 | Shrikant Malviya | SKDU | 0.8292 | 0.4864 |
8 | Nitiz Khanal | Nitiz | 0.8152 | 0.4193 |
9 | Omar Nasr | OAR | 0.7996 | 0.2726 |
10 | Hector | RoVIT | 0.759 | 0.4222 |
Leaderboard-Subtask
Leaderboard for Task-A Scores
S.No | Name | Team Name | Scores |
---|---|---|---|
1 | Suril | SeeTrails | 0.8334 |
2 | Duong Anh Kiet | dakiet | 0.833 |
3 | TSAN-TSUNG | NYCU | 0.8329 |
4 | Shaurya | random.py | 0.8326 |
5 | Xiaoyu | Xiaoyu | 0.8316 |
6 | Raghad_Khalid | TAHAKOM | 0.8305 |
7 | Shrikant Malviya | SKDU | 0.8292 |
8 | Nitiz Khanal | Nitiz | 0.8152 |
9 | Omar Nasr | OAR | 0.7996 |
10 | Hector | RoVIT | 0.759 |
Leaderboard for Task-B Scores
S.No | Name | Team Name | Scores |
---|---|---|---|
1 | Suril | SeeTrails | 0.4986 |
2 | Shaurya | random.py | 0.4936 |
3 | Duong Anh Kiet | dakiet | 0.4935 |
4 | TSAN-TSUNG | NYCU | 0.491 |
5 | Xiaoyu | Xiaoyu | 0.4888 |
6 | Shrikant Malviya | SKDU | 0.4864 |
7 | Raghad_Khalid | TAHAKOM | 0.4816 |
8 | Hector | RoVIT | 0.4222 |
9 | Nitiz Khanal | Nitiz | 0.4193 |
10 | Omar Nasr | OAR | 0.2726 |
IMPORTANT DATES
- 22 October 2024: Release of the training set.
- 10 November 2024: Release of the test set.
- 2 December 2024: Release of the Updated test set.
30 November14 December 2024: Deadline for submitting the final results.- 20 December 2024: Announcement of the results.
12 January16 January 2025: System paper submission deadline (All teams are invited to submit a paper).- 20 January 2025: Notification of system papers.
- 25 January 2025: Camera-ready submission.